Apptica Talks. Episode #1 S2. In-app traffic, fraud, predictive algorithms and future of programmatic advertising with Genrikh Lukianchuk from Bidease.
Kicking off the second season with an episode where Apptica Talks about in-app traffic, fraud, predictive algorithms and future of programmatic advertising with Genrikh Lukianchuk, General Manager, Europe at Bidease.
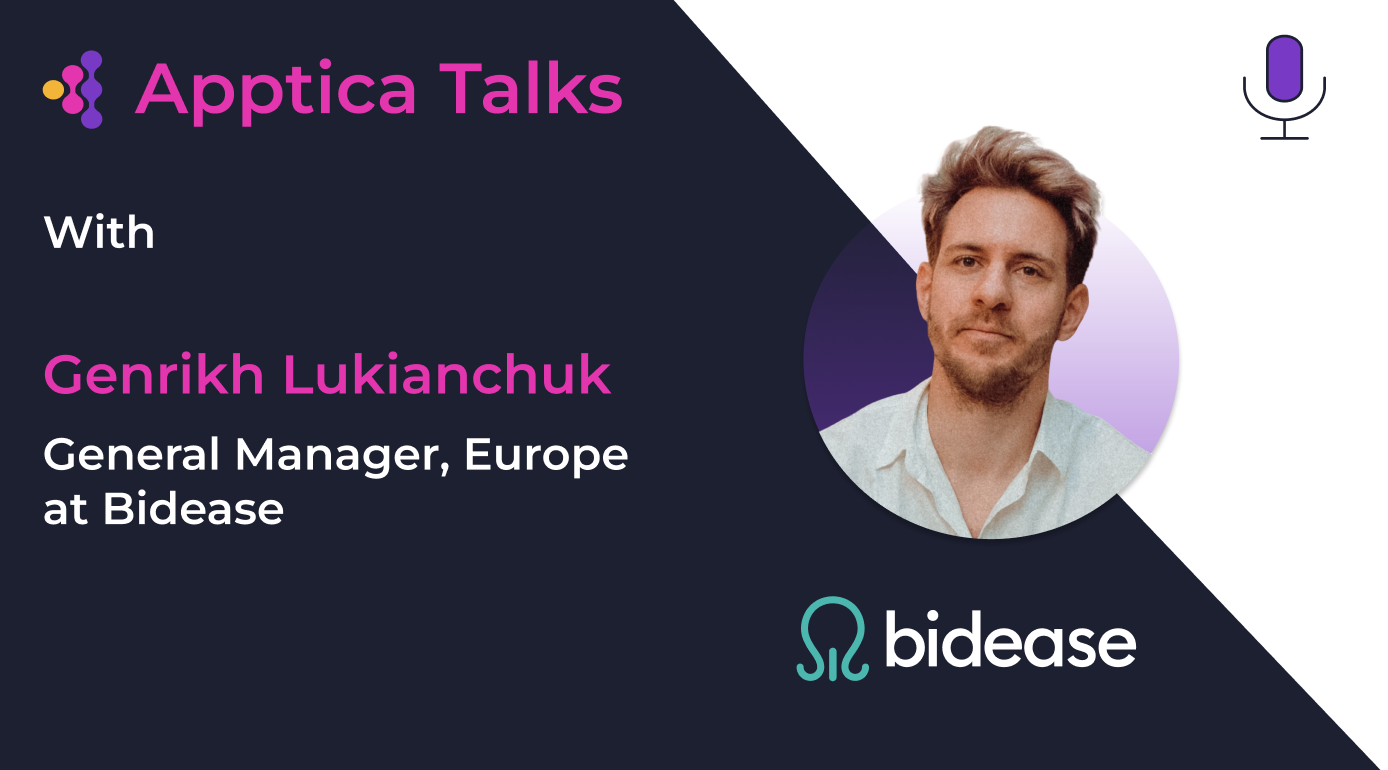
🕺 Kicking off the second season with an episode where Apptica Talks about in-app traffic, fraud, predictive algorithms and future of programmatic advertising with Genrikh Lukianchuk, General Manager, Europe at Bidease
Bidease is a mobile DSP that provides advertisers with access to the entire mobile ecosystem through the world’s most popular mobile publishers and exchanges.
What we have talked about:
- In-app traffic as an exceptional source of advertising
- Fraud: the main channels, ways of detection, current scope, the due diligence to follow
- Effectiveness of programmatic buying: AI and ML
- Emerging trends and technologies in programmatic advertising and mobile industry
Key Takeaways
- In-app traffic is an exceptional source of advertising for various reasons:
- Mobile users are spending 90% of their time on apps, equivalent to more than three hours a day. Thus, apps represent a significant portion of user’s time and attention on daily basis
- In-app ads also have a customer conversion rate that is three times higher than traditional ads and a user retention rate that is four times that of traditional ads
- When a mobile user is browsing websites, advertisers and publishers can only accumulate data about them using cookies which is quite limited. However, when using an application, ad publishers can collect more targeted information through device ID including age, internet provider, gender, operating system, device type, geolocation, and more.
- If a user has installed an ad blocker, it is likely they won't see banner ads. That's not a big concern for in-app advertisers because of the freemium business model which allows them to serve ads to the mobile users who can use the app without paying any price.
2. Wherever the money is – fraud will likely be there. Fraud is a reality of adtech business as an approximate 1$ out of every $3 is lost to this kind of activity, according to IAB statistics.
3. In terms of markets the most impacted are the US due its highest levels of mobile penetration and mobile usage, followed by China, Japan and the UK - together they represent 60%+ of all lost spend due to fraudulent advertising, according to Juniper Research.
4. When speaking about the volumes the trend is on a rise too - YoY lost spend is increasing by 36% on average: just to compare in 2021 industry lost 50$ billion in fraudulent advertising and is estimated to lose 95$ billion this year.
5. The fraudsters operate within various channels, there is no dominant one: device farm, bots, click injection, SDK spoofing.
6. Fraudulent activities in gaming vertical intensified in 2020 when the COVID hit
7. It’s important to have a due diligence of a potential partner (DSP or SSP). A highly professional UA specialist would ask him-/herself the following questions:
- What are the average industry KPIs for his particular vertical or subvertical? What should be considered the base benchmarks?
- What traffic quality standards & practices does a particular DSP/SSP implement? For example, Bidease heavily invests in 2 large areas of traffic quality protection: pre-bid, before we even bid on a particular request, and post-bid, which includes retroactive action on the fraudulent traffic.
- What is their market reputation? Have other companies experienced significant fraud activity coming from the partner in the past?
- What is their billing model? Almost all of Tier 1 SSPs/DSPs operate on CPM model exclusively as working on CPI/CPA billing can launch a “vicious” cycle of sourcing fraudulent activities to achieve contractual KPIs and make “quick buck” by providing extremely low costs on face value to boost their margins.
8. There should be a filigree balance between ML models and human intervention - as relying purely on machine algorithms can lead to significant overfitting problems in the long run. Ideally, good models should have minimal values for errors - leaving room only for so-called base error or due to randomness in the data. It is unrealistic to assume that a person's behavior would be identical on a daily basis and his patterns would not change.
9. Trends to keep an eye on:
- Deep Learning ROAS Optimizations to deal with data sparsity and require less time to learn and optimize faster
- Open AI for Creatives. Dynamically creating user-specific creatives in real time to drive conversions - however, unless the mechanism of approvals is solved it would be a challenge for Clients to opt-in such systems
- Market Price Models to determine fair market value of a bid without 2nd price auctions
You can learn more about Bidease on the website and get more insights in its blog.
🎧 Put on headphones and enjoy:
Spotify
Google Podcast
Apple Podcast
YouTube